
How AI is reducing the risk of fire in the paper sector
The risk of fire is very high in the paper industry – ultimately the materials in this sector are highly flammable. An innovative defect and residual current monitoring solution allows for potential ignition sources to be detected early.
Challenge: flammable material, huge concentration of risk
The paper production and processing industry has always been exposed to high fire risks. Malfunctions of the electrical systems processing the paper are a common cause of fire. At the same time, there are high concentrations of fire loads, such as where used paper or manufactured paper rolls are stored. Insulation faults between the output of frequency converters and the motor of an overhead crane, for example, pose an especially significant risk. In the past, fires in the paper sector have meant high insurance losses. This in turn has led to rising premiums and excesses, especially for claims-heavy contracts. In addition, insurers sometimes require binding risk reduction plans in order to continue providing cover. ‘Suitable fire concepts, such as for high-bay racking with a high potential for large-scale fire, are very demanding and cost-intensive,’ says Manuel Zimmermann, Beyond Insurance Manager at Funk. ‘Cost drivers here include the high operating and maintenance costs, disruptions to operational processes caused by protective devices and unexplained malfunctions. Even if a fire does not occur, faults in electrical systems or protective devices often lead to business interruptions.’
‘Even if a fire does not occur, faults in electrical systems or protective devices often lead to business interruptions.’
Solution: recognising faults before they occur
In collaboration with its partner twingz, Funk offers a client from the paper sector a software solution that permanently monitors certain flow parameters – in this case electrical current – and intelligently analyses the results. The data is recorded in real-time using a special sensor infrastructure: RCM (residual current monitor) sensors measure leakage and residual currents, while PQM (power quality management) systems monitor active energy, voltage, frequency and jitter. The electrical current data is continuously analysed by the predictive deep learning analysis software on the basis of artificial intelligence.
Artificial Intelligence (AI)
AI is an umbrella term for software-based applications that replicate the intelligent performance of the human brain – learning, making judgement calls and solving problems. It is essentially based on algorithms for calculating probabilities and detecting patterns in extensive datasets. The most important feature of AI methods is the ability to learn, whereby the application not only makes decisions based on prescribed, memorised examples it has been fed, but is also able to generalise knowledge gained and apply it correctly in practice. Thanks to these methods, AI applications are constantly improving over time and performing the tasks they have been assigned better and better.
The system looks for abnormalities and patterns that indicate upcoming faults or the risk of a short circuit. This approach means that company personnel are informed early and automatically when there are abnormalities.
Benefit: a problematic industry becomes insurable again
In the described example, the system, which was used as part of Funk Beyond Insurance, contributes to innovative, proactive fire protection. The risk of fire, which is especially critical and typical for the industry, can thus be reduced.
‘Personnel can take action before a costly, large-scale claim arises,’ says Dr Alexander Skorna, Head of Business Development at Funk. ‘In the future, this offers the chance to work with certification bodies and insurers to make affected infrastructures insurable.’ Furthermore, the project is a starting point for creating a technical infrastructure on which the implementation of parametric insurance products can be based.
Parametric Insurance
In the case of parametric insurance, the insurer adjusts claims based on predefined parameters rather than later damage amounts. If a contractually agreed value range is breached as a result of an event, this ‘trigger’ automatically results in an assigned claims payment in response. Any potential deviation of the claims payment from the actual amount of damage incurred remains as the ‘basic risk’. The increasing use of modern sensors and highly developed analysis software has facilitated the constantly improved recording of damage-relevant data flows on the one hand and the development of more reliable models for correlating trigger events and damage amounts on the other.
Health and safety in the workplace also increases. What’s more, early prediction of possible faults and defects can help improve the maintenance process. As a result, not only are the risk and intensity of unplanned downtime reduced, but the frequency and duration of planned downtime (e.g. maintenance and testing) can also be optimised. Overall, costly business interruptions are reduced, while at the same time, the availability of production capacities increases. A decisive factor in this is that maintenance intervals can be controlled in line with needs and cost-effectively.
Predictive Maintenance
Predictive maintenance describes the following concept: unplanned downtime caused by wear and tear or faults is reallocated to planned, needs-based maintenance measures. Suitable sensors detect parameters that can be used to draw conclusions about wear and tear or potential faults – for example, a sensor might track the temperature of a tool, vibrations of an assembly or the start-up time of a motor. Characteristic patterns in this data can be linked to specifically expected damage through suitable models.
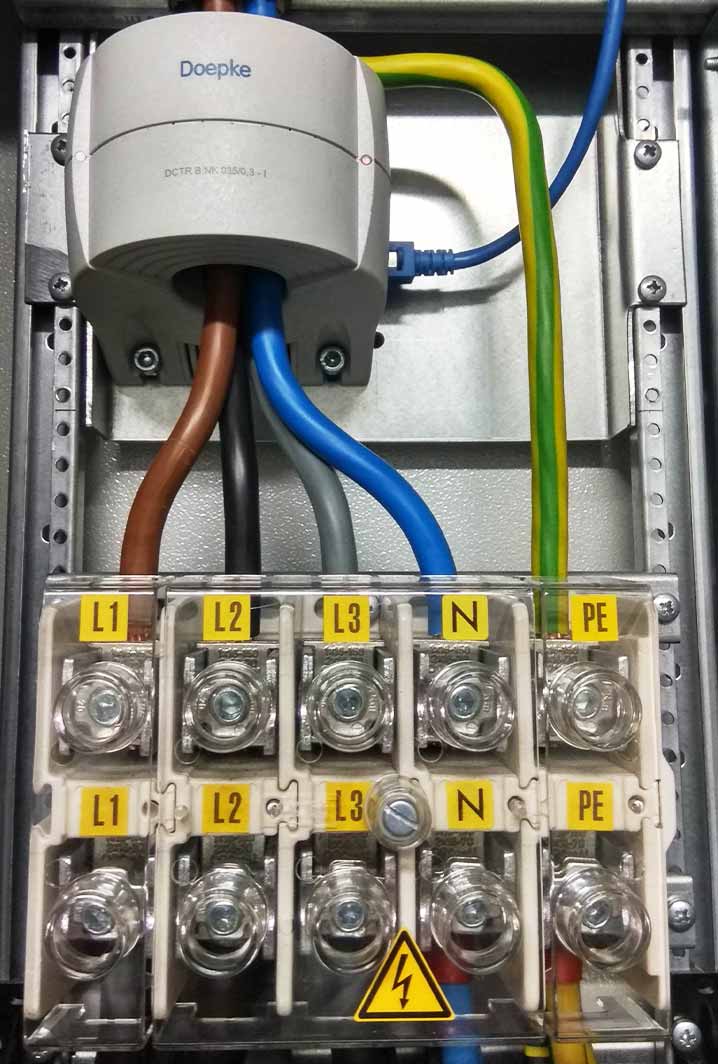
RCM sensors (in grey in the picture) measure leakage and residual currents directly on the cable lines. © twingz
The solution can be used in many areas. The respective consumption can be read off precisely from the electricity data; what’s more, the software solution can be integrated in existing business intelligence systems. This results in extra added value, such as in the area of sustainable energy management.
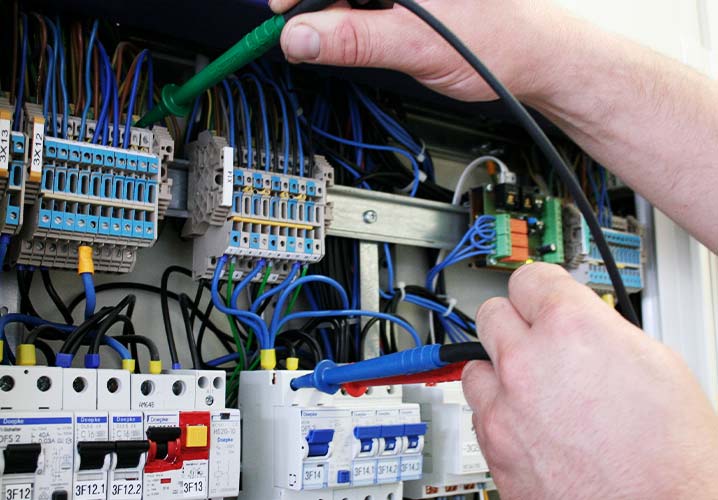
The control cabinets are one of the places where the intelligent software solution monitors and evaluates data. © twingz
Project progress: achieving the objective step by step
The project was divided into phases. This way the approach can be assessed early and the project can be cancelled if prospects do not look good, which reduces the risk. In each phase, the feasibility of the next phase is assessed and the result of this is conveyed to the client.
1. Precise definition of the project scope
2. Definition of the protective aim
3. Definition of the areas and systems to be monitored
4. Presentation of the benefit of the system and creation of the offer
5. Installation and commissioning of the required sensors and integration of the components in the analysis software
6. Start of the teach-in phase (2–6 months depending on the scope), regular adjustment of the key parameters
7. Full operation after teach-in phase
Suitable for: warehouses, data centres and production areas
The software system offered by Funk and its partner twingz can be used to analyse flow parameters: primary current and also water flows. At the heart of the system lie AI-based predictive analytics cloud services combined with a separate sensor infrastructure.
Cloud Computing
Cloud computing describes the approach of providing IT infrastructure (e.g. servers, databases, network components or software) via the internet so that these do not use up resources such as memory space and processing power on the user’s local computer. Such services can usually be accessed dynamically ‘on the cloud’ as needed and be charged for on a per-use basis. Falling back to cloud computing therefore corresponds to classic outsourcing in terms of providing, installing and supporting a company’s own IT infrastructures.
With this approach, companies receive processed data on energy or water consumption as well as risk behaviour patterns and harmful end devices. For example, in the housing industry, the software identifies leaks in water pipes early, so that high consequential damage can be avoided. Likewise, in the production and logistics sectors, potential short circuits and faulty electrical systems can be identified early, allowing for proactive fire protection and increased availability of production capacities. The application fields range from high bay warehouses and data centres to animal stalls and hot and cold production in the food industry.
Your point of contact
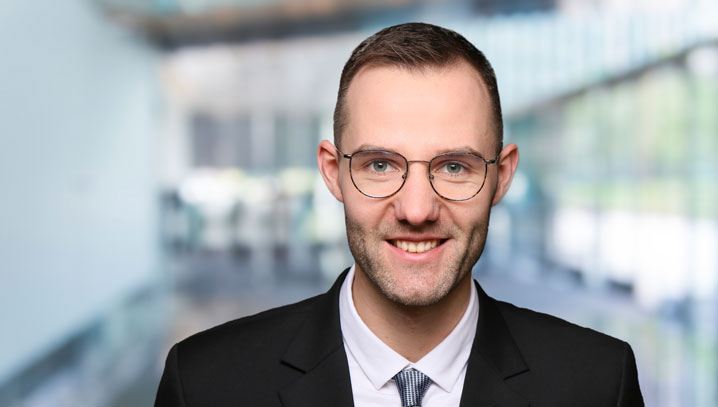
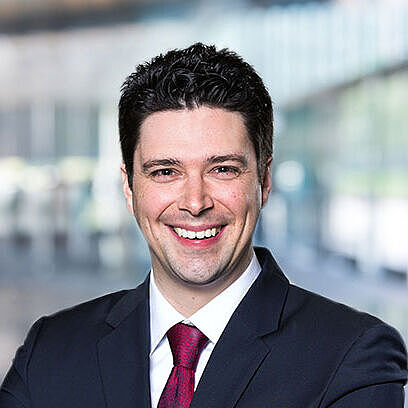
Technical aspects
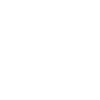
Electrical cables
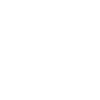
Sensors
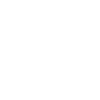
Artificial intelligence
Benefits/objectives
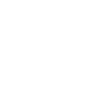
Risk reduction
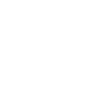
Security and resilience
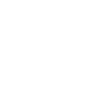
Preventative maintenance